May 14, 2025
Drug Discovery and Recent Advancements in Neurology Centering Bioinformatics
“Neurodiscovery AI has been instrumental in transforming real-world neurology data into actionable insights that improve patient care and sustain private practice. Their commitment to neurologists—ensuring both business success and innovation in drug discovery—makes them an invaluable partner to NeuroNet and the entire field of community neurology.”
Joseph V. Fritz PhDPartner,
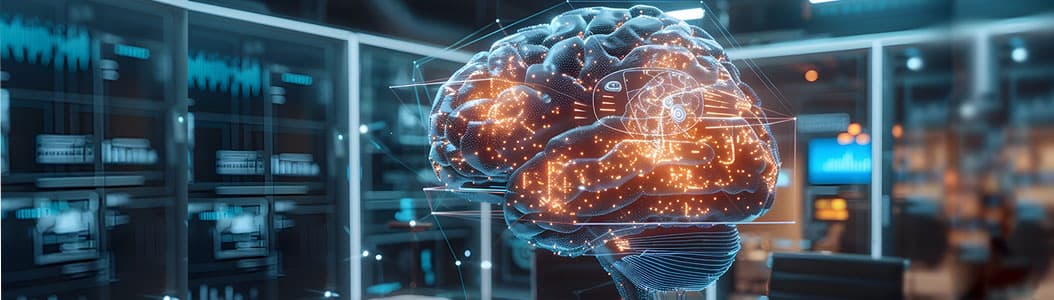
Introduction
Neurological disorders, including Alzheimer’s disease (AD), Parkinson’s disease (PD), multiple sclerosis (MS), amyotrophic lateral sclerosis (ALS), and Huntington’s disease (HD), represent some of the most pressing challenges in modern medicine. These diseases are characterized by progressive degeneration of the nervous system, leading to cognitive decline, motor impairment, and loss of independent function. Despite extensive research, effective treatments remain limited, largely due to the complex and multifactorial nature of these conditions.
Neurological disorders impose a significant global health burden, affecting millions of individuals and their families. With aging populations worldwide, the prevalence of these conditions is rising, intensifying the need for innovative therapeutic strategies. Traditionally, drug discovery in neurology has relied on small-molecule screening, animal models, and clinical trials, but success rates have been low due to the intricate pathophysiology of brain disorders. Many of these diseases involve genetic, epigenetic, environmental, and metabolic components, making them particularly difficult to treat. Moreover, the blood-brain barrier (BBB) poses an additional challenge by restricting drug penetration into the central nervous system (CNS), thereby limiting the effectiveness of many therapeutic compounds.
Recent advances in bioinformatics, particularly in the field of multi-omics integration, have provided new insights into the molecular mechanisms underlying neurological disorders. The ability to analyze large-scale genomic, transcriptomic, proteomic, and metabolomic data has revolutionized drug discovery efforts, enabling researchers to identify novel drug targets and develop precision medicine approaches. With the integration of artificial intelligence (AI) and machine learning (ML), bioinformatics-driven drug discovery is accelerating at an unprecedented pace, offering hope for more effective therapies.
This article explores the role of bioinformatics in neurological drug discovery, with a focus on multi-omics data integration, AI-driven methodologies, and recent breakthroughs in the field.
The Role of Bioinformatics in Neurological Drug Discovery
Bioinformatics plays a crucial role in drug discovery by enabling high-throughput data analysis, biomarker identification, and in silico drug screening. The key applications of bioinformatics in neurology include:
1. Multi-Omics Data Integration
The field of multi-omics refers to the integration of various biological data types, including genomics, transcriptomics, proteomics, metabolomics, and epigenomics, to provide a comprehensive understanding of disease mechanisms. Each of these omics layers offers unique insights:
-
Genomics focuses on identifying genetic variations, such as single nucleotide polymorphisms (SNPs) and copy number variations (CNVs), that contribute to disease susceptibility. Genome-wide association studies (GWAS) have uncovered numerous genetic risk factors for neurological diseases, such as the APOE4 allele in Alzheimer’s disease and LRRK2 mutations in Parkinson’s disease. Advancements in whole-genome sequencing have provided deeper insights into rare mutations that may contribute to disease pathology.
-
Transcriptomics examines gene expression patterns to understand how genetic alterations influence disease progression. RNA sequencing (RNA-seq) studies have revealed dysregulated pathways in neurodegeneration, including inflammatory and metabolic pathways that contribute to disease onset. Single-cell RNA sequencing (scRNA-seq) has further allowed researchers to study gene expression at the level of individual neurons and glial cells, providing unprecedented insights into cellular heterogeneity in neurological diseases.
-
Proteomics analyzes protein expression and modifications, shedding light on post-translational changes that affect cellular function. Mass spectrometry-based proteomics has been instrumental in identifying misfolded proteins and aggregation-prone peptides, such as beta-amyloid in AD and alpha-synuclein in PD. Additionally, recent advancements in cryo-electron microscopy (cryo-EM) have allowed for high-resolution visualization of neurotoxic protein aggregates, enabling the design of novel inhibitors targeting protein misfolding.
-
Metabolomics investigates small-molecule metabolites that serve as indicators of cellular activity and disease states. Metabolic profiling has provided insights into mitochondrial dysfunction in neurodegenerative diseases, helping identify potential metabolic targets for drug development. For example, alterations in the kynurenine pathway have been implicated in neuroinflammation and neurodegeneration, making it a promising therapeutic target.
-
Epigenomics examines heritable changes in gene expression that do not alter the underlying DNA sequence but play a crucial role in neurological disorders. DNA methylation, histone modifications, and non-coding RNAs are key epigenetic regulators influencing neurodegenerative disease pathways. Studies have shown that altered DNA methylation patterns in AD and PD patients affect genes involved in neuroinflammation and synaptic plasticity. Recent research has suggested that targeting epigenetic modifications using small-molecule inhibitors or gene-editing techniques may offer novel therapeutic strategies for neurological diseases.
By integrating these multi-omics datasets, researchers can construct comprehensive molecular networks that reveal interactions between genes, proteins, and metabolites in neurological disorders. Multi-omics studies have identified key molecular pathways, such as neuroinflammation, oxidative stress, and protein aggregation, which contribute to neurodegenerative diseases. Systems biology approaches enable researchers to develop holistic disease models that can guide drug discovery and biomarker identification.
2. Artificial Intelligence and Machine Learning
Machine learning (ML) and deep learning (DL) models are increasingly being used to predict disease progression, classify patients based on molecular signatures, and identify novel drug targets. Neural networks trained on large-scale datasets from consortia like the AMP-PD and ADNI provide insights into disease subtypes and progression patterns.
One major breakthrough is the application of AI in drug-target interaction prediction. By utilizing deep learning models, researchers can screen vast chemical libraries against known neurological disease targets with remarkable accuracy. AI-driven approaches have also facilitated structure-based drug design, enhancing the discovery of small molecules with high binding affinities to disease-associated proteins.
3. Computational Drug Repurposing
In silico drug repurposing leverages bioinformatics approaches such as connectivity mapping, network pharmacology, and docking simulations to identify existing drugs that may be effective for neurological diseases. For instance, AI-based screening has identified drugs like nilotinib and ambroxol as potential candidates for Parkinson’s disease.
Computational drug repurposing methods also leverage transcriptomic and proteomic signatures to match disease-specific molecular profiles with existing pharmacological compounds. Large-scale drug repurposing initiatives, such as Open Targets and the Library of Integrated Network-Based Cellular Signatures (LINCS), provide valuable resources for identifying candidate drugs.
4. Biomarker Discovery for Precision Medicine
Biomarker discovery using bioinformatics has led to the identification of novel diagnostic and prognostic markers. For example, cerebrospinal fluid (CSF) and blood-based biomarkers such as neurofilament light chain (NfL) have been validated using proteomics and machine learning approaches to assess disease progression in MS and AD.
Recent advancements in multi-omics data integration have enabled the discovery of novel biomarkers that may aid in early disease detection and personalized treatment strategies. For example, proteogenomic approaches combining proteomics and genomics have revealed disease-specific protein signatures in AD, paving the way for precision neurology.
Conclusion
Bioinformatics has transformed the landscape of neurological drug discovery by enabling data-driven insights into disease mechanisms, accelerating target identification, and enhancing drug repurposing strategies. With continuous advancements in AI, genomics, and systems biology, the future of drug discovery using AI in neurology holds great promise for developing effective, personalized treatments for devastating brain disorders.
References
- Zhang, B., Gaiteri, C., Bodea, L. G., et al. (2013). Integrated systems approach identifies genetic nodes and networks in late-onset Alzheimer's disease. Cell, 153(3), 707-720.
- Hendershott, T. R., & Guissart, C. (2021). Advances in epigenetic mechanisms and drug discovery for neurodegenerative diseases. Trends in Pharmacological Sciences, 42(8), 623-637.
- Wang, M., Roussos, P., McKenzie, A., et al. (2016). Integrative network analysis of nineteen brain regions identifies molecular signatures and pathways linked to neurodegenerative diseases. Neuron, 92(4), 939-951.
- Mathys, H., Davila-Velderrain, J., Peng, Z., et al. (2019). Single-cell transcriptomic analysis of Alzheimer's disease. Nature, 570(7761), 332-337.